티스토리 뷰
본문을 통해 ICP 적용분야 및 알고리즘 흐름에 대한 간략한 내용을 다뤄보려고 한다.
ICP
- ICP란 Iteratvie Closest Point의 줄임말로, 서로 다른 두 개 점군의 정합(Registration)과정을 통해 변환행렬(Transform)을 구하는 대표적인 알고리즘이다.
- 알고리즘의 핵심은 Nearest Neighborhood 알고리즘을 통해 가장 근접한 점군(closest point)을 매칭하고, Leaset Squares Method를 이용하여 정합오차(registration error)를 최소화하는 transform을 구하게 됩니다.
- Iterative(Least Square Method) + Closest Point(Nearest Neighborhood)여서 이름이 ICP가 아닐까 싶네요ㅎㅎ
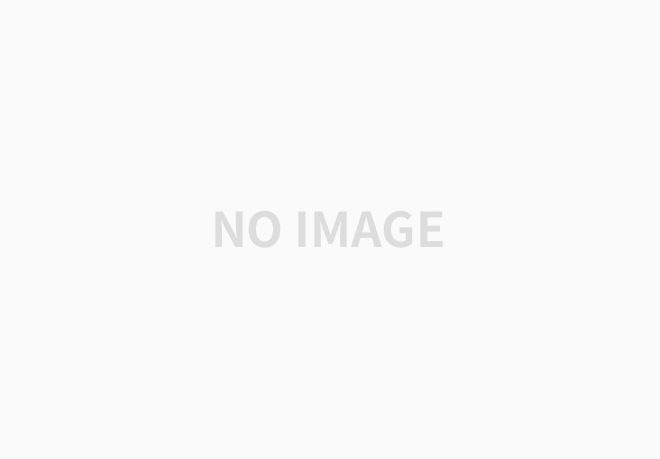
적용 분야
- Visual SLAM, LiDAR SLAM에서 많이 사용되는 알고리즘이다.
- SLAM의 Loop Closure 노드에서 폐쇄루트가 감지되면 전역적으로 그래프 최적화 알고리즘에도 적용 될 수 있다.
- Localization 분야에서, ICP를 통해 LaserOdometry를 구할 수도 있으며, 기존 wheel odometry에서 생길 수 있는 wheel slippage 현상을 ICP를 통해 보정하는데에도 사용 할 수 있다.
- 개인적인 생각으로는 모바일 로봇의 Localization에서 만이 아닌 Humanoid, 혹은 Legged Robot의 Localization방법으로 ICP를 활용하는 것도 괜찮을 것 같다.
알고리즘 구현
ICP를 통해 구 점군간의 transform은 다음과 같은 단계로 이루어진다.
- 두 Pointcloud의 point들 간의 matching을 결정한다.
- 정합오차를 최소화하는 transform을 구하여 이전에 관측된 데이터를 transform 한다.
코드 분석
Clay Flannigan이 구현한 코드를 이용해 알고리즘을 분석해 본다.
def icp(A, B, init_pose=None, max_iterations=20, tolerance=0.001):
assert A.shape == B.shape
m = A.shape[1]
src = np.ones((m+1,A.shape[0]))
dst = np.ones((m+1,B.shape[0]))
src[:m,:] = np.copy(A.T)
dst[:m,:] = np.copy(B.T)
# apply the initial pose estimation
if init_pose is not None:
src = np.dot(init_pose, src)
prev_error = 0
for i in range(max_iterations):
distances, indices = nearest_neighbor(src[:m,:].T, dst[:m,:].T)
T,_,_ = best_fit_transform(src[:m,:].T, dst[:m,indices].T)
src = np.dot(T, src)
# check error
mean_error = np.mean(distances)
if np.abs(prev_error - mean_error) < tolerance:
break
prev_error = mean_error
# calculate final transformation
T,_,_ = best_fit_transform(A, src[:m,:].T)
return T, distances,
def best_fit_transform(A, B):
assert A.shape == B.shape
m = A.shape[1]
# translate points to their centroids
centroid_A = np.mean(A, axis=0)
centroid_B = np.mean(B, axis=0)
AA = A - centroid_A
BB = B - centroid_B
# rotation matrix
H = np.dot(AA.T, BB)
U, S, Vt = np.linalg.svd(H)
R = np.dot(Vt.T, U.T)
# special reflection case
if np.linalg.det(R) < 0:
Vt[m-1,:] *= -1
R = np.dot(Vt.T, U.T)
# translation
t = centroid_B.T - np.dot(R,centroid_A.T)
# homogeneous transformation
T = np.identity(m+1)
T[:m, :m] = R
T[:m, m] = t
return T, R, t
알고리즘의 한계
- ICP는 모든 점군에 대해 게산하므로 계산속도에 대한 문제가 존재한다, 즉 실시간 처리가 불가능하다.
- 이를 해결하기 위해서는, 모든 점군에 대한 계산을 하는 것이 아닌, 특징되는 점군을 뽑아 처리 속도를 높이는 방법이 있다.
- ORB, SIFT, SURF와 같은 특징점을 추출하여 ICP에 적용한다.
- 혹은 RANSAC, NDT, Octree와 같은 방법들을 활용하는 방법이 있다.
마무리 내 생각
- 지금까지는 ICP를 사용한다고 했을 때, 단순 Localization에서의 기능적으로만 생각을 했었는데, 보다 포괄적으로 사용되는 알고리즘으로 SLAM분야에서는 어떻게 사용되는지에 대한 고찰을 가져보면 좋을 것 같다.
Ref
참고 : 이론
- https://define.tistory.com/entry/Iterative-Closest-Point
- http://daddynkidsmakers.blogspot.com/2021/09/icpiterative-closest-point.html
- https://ieeexplore.ieee.org/document/4767965
참고 : 예제 및 소스코드
댓글
공지사항
최근에 올라온 글
최근에 달린 댓글
- Total
- Today
- Yesterday
링크
TAG
- trapezoidal
- wrench
- git
- optimal control
- Screw
- ICP
- lqr control
- Modern Robotics
- manipulator
- odometry
- NumericalComputation
- Mobile Robot
- Jacobian
- LaTeX
- Forward Kinematics
- Slam
- ORB
- Kinematics
- Twists
- inverse kinematics
- repeatability
- PID CONTROL
- odom
- Localization
- Robotics
- control
- visual slam
- paper review
- trajectory planning
- Configuration Space
일 | 월 | 화 | 수 | 목 | 금 | 토 |
---|---|---|---|---|---|---|
1 | 2 | 3 | 4 | 5 | ||
6 | 7 | 8 | 9 | 10 | 11 | 12 |
13 | 14 | 15 | 16 | 17 | 18 | 19 |
20 | 21 | 22 | 23 | 24 | 25 | 26 |
27 | 28 | 29 | 30 |
글 보관함